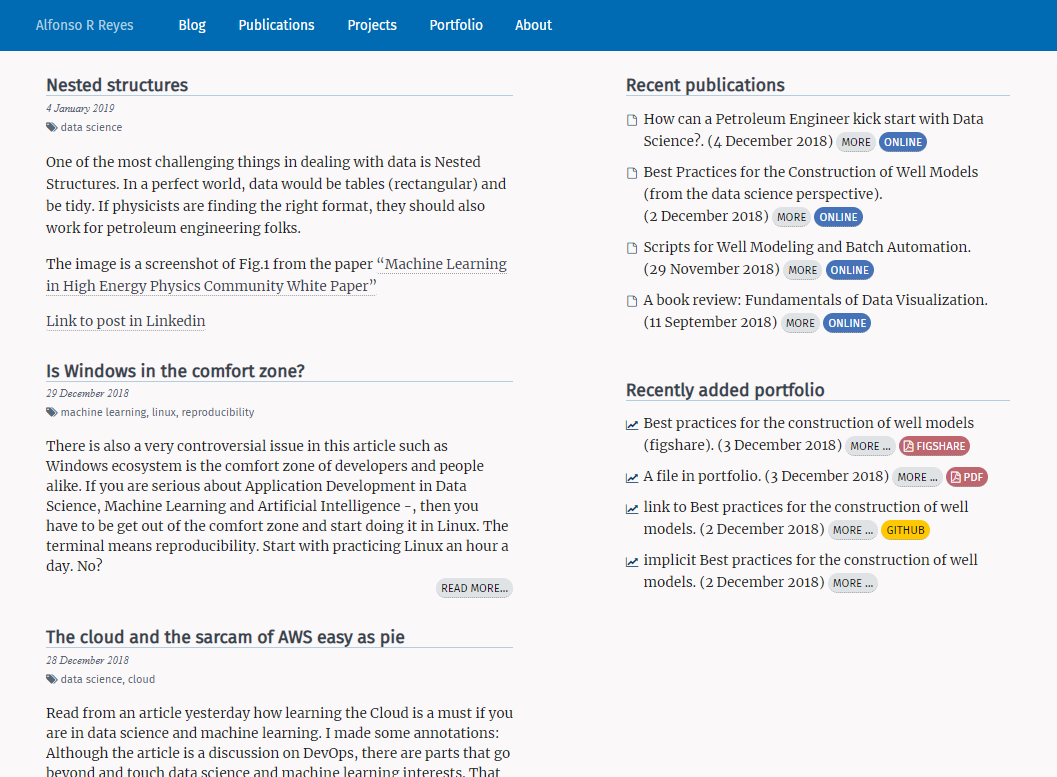
Introduction
As I announced last week, my blog is now online at http://blog.oilgainsanalytics.com. LinkedIn may obfuscate the link so I am also providing it as an image below. Clicking on the image will bring you to the blog:
blog.oilgainsanalytics.com
or
oilgainsanalytics.com/blog
Motivation
I believe in the sharing philosophy of data science as I learned it from my biostatistician instructors at Johns Hopkins University (Peng, Leek, Caffo, et al). There are so many career-changing lessons that you could pick up with these professors at Coursera. Data Science is a whole multidisciplinary, or should I say interdisciplinary as in Wikipedia, field that combines statistics, mathematics, computer science, and data engineering that gives you the power to dig deep into your data and make astonishing discoveries to bring real value to your organization.
I felt the need of an online website that could support Rmarkdown instead of copying and pasting code and pictures to [LinkedIn][3] every time.
Under the hood
This blog uses the R package blogdown, an application that converts markdown and Rmarkdown to Html and Javascript, making easier for me to run code and publish it immediately as it exactly is written.
One of the articles I am preparing on multiwell statistics
-coming in few days-, will be written in Python and R together using RStudio IDE1. It will be about running statistical methods on datasets originated by batch automation produced by communicating with the production optimization software Prosper via OpenServer, work that I developed few years ago but very relevant nowadays.
Still, I will continue publishing in LinkedIn but the extended, best and more current version of the post running with R and Python code will be in the blog.
A quick demonstration
This first example assumes you installed a Portable Python WinPython 3.7.1, 64-bit, under your user directory in Windows. Not to worry, I will explain in a separate set of slides how to get Python installed.
Loading Python in RStudio
First, we load a Python environment. In this case, I am loading the Python portable version I mentioned above, but it could be Anaconda or another flavor. I have found that WinPython is just much easier to navigate and make it work.
This is the code that load the Pythobn environment that will together with R.
# load the package that makes R and Python talk
library(reticulate)
# set the preferred Python to execute
user_profile <- Sys.getenv("USERPROFILE") # user folder
# python_portable <- normalizePath(file.path(user_profile, # Python location
# "WPy-3710zero/python-3.7.1.amd64/python.exe"))
# reticulate::use_python(python_portable, required = TRUE)
# find out if it took it
reticulate::py_config()
#> python: /usr/bin/python3
#> libpython: /usr/lib/python3.8/config-3.8-x86_64-linux-gnu/libpython3.8.so
#> pythonhome: //usr://usr
#> version: 3.8.10 (default, Sep 28 2021, 16:10:42) [GCC 9.3.0]
#> numpy: /usr/lib/python3/dist-packages/numpy
#> numpy_version: 1.17.4
#>
#> python versions found:
#> /usr/bin/python3
#> /usr/bin/python
Example 1
Produce a simple Python matplotlib plot.
This is not easy as it seems using other flavors of Python. I found that WinPython makes this plotting task easier for beginners or advanced levels. But this should be your test of fire because it uses several Python packages: matplotlib
, numpy
, PyQt
, etc. So, if this works, almost everything else will work.
# a simple sine plot
import matplotlib.pyplot as plt
import numpy as np
t = np.arange(0.0, 2.0, 0.01)
s = 1 + np.sin(2*np.pi*t)
plt.plot(t, s)
plt.xlabel('time (s)')
plt.ylabel('voltage (mV)')
plt.title('About as simple as it gets, folks')
plt.grid(True)
plt.savefig("sine.png")
plt.show()
I know. This can be done easily in Jupyter notebooks but the objective here is to mix the power of Python and R running in a native R platform. I will explain later why.
Why the Python plots if R has ggplot2
One big reason: doing some data science and machine learning with reservoir blocks will require some 3d capabilities and Python has packages available for that. R also does but it would take more work. Refer the next example.
Example 2
Now, we are going to plot a pretended reservoir block for reservoir simulation. We are going to need it at sometime to build the machine learning algorithm and a artificial intelligence agent to do, let’s say, history matching, for starters. I found this beautiful example in the matplotlib
tutorial section.
# https://matplotlib.org/api/_as_gen/mpl_toolkits.mplot3d.axes3d.Axes3D.html
#=======================================================
#3D voxel / volumetric plot with cylindrical coordinates
#=======================================================
# Demonstrates using the ``x, y, z`` arguments of ``ax.voxels``.
import matplotlib.pyplot as plt
import matplotlib.colors
import numpy as np
# This import registers the 3D projection, but is otherwise unused.
from mpl_toolkits.mplot3d import Axes3D # noqa: F401 unused import
def midpoints(x):
sl = ()
for i in range(x.ndim):
x = (x[sl + np.index_exp[:-1]] + x[sl + np.index_exp[1:]]) / 2.0
sl += np.index_exp[:]
return x
# prepare some coordinates, and attach rgb values to each
r, theta, z = np.mgrid[0:1:11j, 0:np.pi*2:25j, -0.5:0.5:11j]
x = r*np.cos(theta)
y = r*np.sin(theta)
rc, thetac, zc = midpoints(r), midpoints(theta), midpoints(z)
# define a wobbly torus about [0.7, *, 0]
sphere = (rc - 0.7)**2 + (zc + 0.2*np.cos(thetac*2))**2 < 0.2**2
# combine the color components
hsv = np.zeros(sphere.shape + (3,))
hsv[..., 0] = thetac / (np.pi*2)
hsv[..., 1] = rc
hsv[..., 2] = zc + 0.5
colors = matplotlib.colors.hsv_to_rgb(hsv)
# and plot everything
fig = plt.figure()
ax = fig.gca(projection='3d')
ax.voxels(x, y, z, sphere,
facecolors=colors,
edgecolors=np.clip(2*colors - 0.5, 0, 1), # brighter
linewidth=0.5)
#> {(5, 0, 2): <mpl_toolkits.mplot3d.art3d.Poly3DCollection object at 0x7fe9efbc3a30>, (5, 0, 3): <mpl_toolkits.mplot3d.art3d.Poly3DCollection object at 0x7fe9efbc3c70>, (5, 1, 2): <mpl_toolkits.mplot3d.art3d.Poly3DCollection object at 0x7fe9efbc3b80>, (5, 1, 4): <mpl_toolkits.mplot3d.art3d.Poly3DCollection object at 0x7fe9efb76160>, (5, 2, 3): <mpl_toolkits.mplot3d.art3d.Poly3DCollection object at 0x7fe9efb763d0>, (5, 2, 5): <mpl_toolkits.mplot3d.art3d.Poly3DCollection object at 0x7fe9efb76640>, (5, 3, 4): <mpl_toolkits.mplot3d.art3d.Poly3DCollection object at 0x7fe9efb768b0>, (5, 3, 6): <mpl_toolkits.mplot3d.art3d.Poly3DCollection object at 0x7fe9efb76b20>, (5, 4, 5): <mpl_toolkits.mplot3d.art3d.Poly3DCollection object at 0x7fe9efb76d90>, (5, 4, 7): <mpl_toolkits.mplot3d.art3d.Poly3DCollection object at 0x7fe9efb76f40>, (5, 5, 6): <mpl_toolkits.mplot3d.art3d.Poly3DCollection object at 0x7fe9efb862b0>, (5, 5, 7): <mpl_toolkits.mplot3d.art3d.Poly3DCollection object at 0x7fe9efb86520>, (5, 6, 6): <mpl_toolkits.mplot3d.art3d.Poly3DCollection object at 0x7fe9efb86790>, (5, 6, 7): <mpl_toolkits.mplot3d.art3d.Poly3DCollection object at 0x7fe9efb86a00>, (5, 7, 5): <mpl_toolkits.mplot3d.art3d.Poly3DCollection object at 0x7fe9efb86c70>, (5, 7, 7): <mpl_toolkits.mplot3d.art3d.Poly3DCollection object at 0x7fe9efb86ee0>, (5, 8, 4): <mpl_toolkits.mplot3d.art3d.Poly3DCollection object at 0x7fe9efb97190>, (5, 8, 6): <mpl_toolkits.mplot3d.art3d.Poly3DCollection object at 0x7fe9efb97400>, (5, 9, 3): <mpl_toolkits.mplot3d.art3d.Poly3DCollection object at 0x7fe9efb97670>, (5, 9, 5): <mpl_toolkits.mplot3d.art3d.Poly3DCollection object at 0x7fe9efb978e0>, (5, 10, 2): <mpl_toolkits.mplot3d.art3d.Poly3DCollection object at 0x7fe9efb97b50>, (5, 10, 4): <mpl_toolkits.mplot3d.art3d.Poly3DCollection object at 0x7fe9efb97dc0>, (5, 11, 2): <mpl_toolkits.mplot3d.art3d.Poly3DCollection object at 0x7fe9efb97f70>, (5, 11, 3): <mpl_toolkits.mplot3d.art3d.Poly3DCollection object at 0x7fe9efb282e0>, (5, 12, 2): <mpl_toolkits.mplot3d.art3d.Poly3DCollection object at 0x7fe9efb28550>, (5, 12, 3): <mpl_toolkits.mplot3d.art3d.Poly3DCollection object at 0x7fe9efb287c0>, (5, 13, 2): <mpl_toolkits.mplot3d.art3d.Poly3DCollection object at 0x7fe9efb28a30>, (5, 13, 4): <mpl_toolkits.mplot3d.art3d.Poly3DCollection object at 0x7fe9efb28ca0>, (5, 14, 3): <mpl_toolkits.mplot3d.art3d.Poly3DCollection object at 0x7fe9efb28f10>, (5, 14, 5): <mpl_toolkits.mplot3d.art3d.Poly3DCollection object at 0x7fe9efb381c0>, (5, 15, 4): <mpl_toolkits.mplot3d.art3d.Poly3DCollection object at 0x7fe9efb38430>, (5, 15, 6): <mpl_toolkits.mplot3d.art3d.Poly3DCollection object at 0x7fe9efb386a0>, (5, 16, 5): <mpl_toolkits.mplot3d.art3d.Poly3DCollection object at 0x7fe9efb38910>, (5, 16, 7): <mpl_toolkits.mplot3d.art3d.Poly3DCollection object at 0x7fe9efb38b80>, (5, 17, 6): <mpl_toolkits.mplot3d.art3d.Poly3DCollection object at 0x7fe9efb38df0>, (5, 17, 7): <mpl_toolkits.mplot3d.art3d.Poly3DCollection object at 0x7fe9efb38fa0>, (5, 18, 6): <mpl_toolkits.mplot3d.art3d.Poly3DCollection object at 0x7fe9efb49310>, (5, 18, 7): <mpl_toolkits.mplot3d.art3d.Poly3DCollection object at 0x7fe9efb49580>, (5, 19, 5): <mpl_toolkits.mplot3d.art3d.Poly3DCollection object at 0x7fe9efb497f0>, (5, 19, 7): <mpl_toolkits.mplot3d.art3d.Poly3DCollection object at 0x7fe9efb49a60>, (5, 20, 4): <mpl_toolkits.mplot3d.art3d.Poly3DCollection object at 0x7fe9efb49cd0>, (5, 20, 6): <mpl_toolkits.mplot3d.art3d.Poly3DCollection object at 0x7fe9efb49f40>, (5, 21, 3): <mpl_toolkits.mplot3d.art3d.Poly3DCollection object at 0x7fe9efb581f0>, (5, 21, 5): <mpl_toolkits.mplot3d.art3d.Poly3DCollection object at 0x7fe9efb58460>, (5, 22, 2): <mpl_toolkits.mplot3d.art3d.Poly3DCollection object at 0x7fe9efb586d0>, (5, 22, 4): <mpl_toolkits.mplot3d.art3d.Poly3DCollection object at 0x7fe9efb58940>, (5, 23, 2): <mpl_toolkits.mplot3d.art3d.Poly3DCollection object at 0x7fe9efb58bb0>, (5, 23, 3): <mpl_toolkits.mplot3d.art3d.Poly3DCollection object at 0x7fe9efb58e20>, (6, 0, 1): <mpl_toolkits.mplot3d.art3d.Poly3DCollection object at 0x7fe9efb58fd0>, (6, 0, 4): <mpl_toolkits.mplot3d.art3d.Poly3DCollection object at 0x7fe9bc64f340>, (6, 1, 2): <mpl_toolkits.mplot3d.art3d.Poly3DCollection object at 0x7fe9bc64f5b0>, (6, 1, 5): <mpl_toolkits.mplot3d.art3d.Poly3DCollection object at 0x7fe9bc64f850>, (6, 2, 3): <mpl_toolkits.mplot3d.art3d.Poly3DCollection object at 0x7fe9bc64fac0>, (6, 2, 5): <mpl_toolkits.mplot3d.art3d.Poly3DCollection object at 0x7fe9bc64fd30>, (6, 3, 4): <mpl_toolkits.mplot3d.art3d.Poly3DCollection object at 0x7fe9bc64ffa0>, (6, 3, 6): <mpl_toolkits.mplot3d.art3d.Poly3DCollection object at 0x7fe9bc65d250>, (6, 4, 4): <mpl_toolkits.mplot3d.art3d.Poly3DCollection object at 0x7fe9bc65d4c0>, (6, 4, 7): <mpl_toolkits.mplot3d.art3d.Poly3DCollection object at 0x7fe9bc65d730>, (6, 5, 5): <mpl_toolkits.mplot3d.art3d.Poly3DCollection object at 0x7fe9bc65d9a0>, (6, 5, 8): <mpl_toolkits.mplot3d.art3d.Poly3DCollection object at 0x7fe9bc65dc10>, (6, 6, 5): <mpl_toolkits.mplot3d.art3d.Poly3DCollection object at 0x7fe9bc65de80>, (6, 6, 8): <mpl_toolkits.mplot3d.art3d.Poly3DCollection object at 0x7fe9bc66e130>, (6, 7, 4): <mpl_toolkits.mplot3d.art3d.Poly3DCollection object at 0x7fe9bc66e3a0>, (6, 7, 7): <mpl_toolkits.mplot3d.art3d.Poly3DCollection object at 0x7fe9bc66e610>, (6, 8, 4): <mpl_toolkits.mplot3d.art3d.Poly3DCollection object at 0x7fe9bc66e880>, (6, 8, 6): <mpl_toolkits.mplot3d.art3d.Poly3DCollection object at 0x7fe9bc66eaf0>, (6, 9, 3): <mpl_toolkits.mplot3d.art3d.Poly3DCollection object at 0x7fe9bc66ed60>, (6, 9, 5): <mpl_toolkits.mplot3d.art3d.Poly3DCollection object at 0x7fe9bc66efd0>, (6, 10, 2): <mpl_toolkits.mplot3d.art3d.Poly3DCollection object at 0x7fe9bc67f280>, (6, 10, 5): <mpl_toolkits.mplot3d.art3d.Poly3DCollection object at 0x7fe9bc67f4f0>, (6, 11, 1): <mpl_toolkits.mplot3d.art3d.Poly3DCollection object at 0x7fe9bc67f760>, (6, 11, 4): <mpl_toolkits.mplot3d.art3d.Poly3DCollection object at 0x7fe9bc67f9d0>, (6, 12, 1): <mpl_toolkits.mplot3d.art3d.Poly3DCollection object at 0x7fe9bc67fc40>, (6, 12, 4): <mpl_toolkits.mplot3d.art3d.Poly3DCollection object at 0x7fe9bc67feb0>, (6, 13, 2): <mpl_toolkits.mplot3d.art3d.Poly3DCollection object at 0x7fe9bc610160>, (6, 13, 5): <mpl_toolkits.mplot3d.art3d.Poly3DCollection object at 0x7fe9bc6103d0>, (6, 14, 3): <mpl_toolkits.mplot3d.art3d.Poly3DCollection object at 0x7fe9bc610640>, (6, 14, 5): <mpl_toolkits.mplot3d.art3d.Poly3DCollection object at 0x7fe9bc6108b0>, (6, 15, 4): <mpl_toolkits.mplot3d.art3d.Poly3DCollection object at 0x7fe9bc610b20>, (6, 15, 6): <mpl_toolkits.mplot3d.art3d.Poly3DCollection object at 0x7fe9bc610d90>, (6, 16, 4): <mpl_toolkits.mplot3d.art3d.Poly3DCollection object at 0x7fe9bc610f40>, (6, 16, 7): <mpl_toolkits.mplot3d.art3d.Poly3DCollection object at 0x7fe9bc61f2b0>, (6, 17, 5): <mpl_toolkits.mplot3d.art3d.Poly3DCollection object at 0x7fe9bc61f520>, (6, 17, 8): <mpl_toolkits.mplot3d.art3d.Poly3DCollection object at 0x7fe9bc61f790>, (6, 18, 5): <mpl_toolkits.mplot3d.art3d.Poly3DCollection object at 0x7fe9bc61fa00>, (6, 18, 8): <mpl_toolkits.mplot3d.art3d.Poly3DCollection object at 0x7fe9bc61fc70>, (6, 19, 4): <mpl_toolkits.mplot3d.art3d.Poly3DCollection object at 0x7fe9bc61fee0>, (6, 19, 7): <mpl_toolkits.mplot3d.art3d.Poly3DCollection object at 0x7fe9bc630190>, (6, 20, 4): <mpl_toolkits.mplot3d.art3d.Poly3DCollection object at 0x7fe9bc630400>, (6, 20, 6): <mpl_toolkits.mplot3d.art3d.Poly3DCollection object at 0x7fe9bc630670>, (6, 21, 3): <mpl_toolkits.mplot3d.art3d.Poly3DCollection object at 0x7fe9bc6308e0>, (6, 21, 5): <mpl_toolkits.mplot3d.art3d.Poly3DCollection object at 0x7fe9bc630b50>, (6, 22, 2): <mpl_toolkits.mplot3d.art3d.Poly3DCollection object at 0x7fe9bc630dc0>, (6, 22, 5): <mpl_toolkits.mplot3d.art3d.Poly3DCollection object at 0x7fe9bc630f70>, (6, 23, 1): <mpl_toolkits.mplot3d.art3d.Poly3DCollection object at 0x7fe9bc6402e0>, (6, 23, 4): <mpl_toolkits.mplot3d.art3d.Poly3DCollection object at 0x7fe9bc640550>, (7, 0, 1): <mpl_toolkits.mplot3d.art3d.Poly3DCollection object at 0x7fe9bc6407c0>, (7, 0, 4): <mpl_toolkits.mplot3d.art3d.Poly3DCollection object at 0x7fe9bc640a30>, (7, 1, 2): <mpl_toolkits.mplot3d.art3d.Poly3DCollection object at 0x7fe9bc640ca0>, (7, 1, 5): <mpl_toolkits.mplot3d.art3d.Poly3DCollection object at 0x7fe9bc640f10>, (7, 2, 3): <mpl_toolkits.mplot3d.art3d.Poly3DCollection object at 0x7fe9bc5d31c0>, (7, 2, 5): <mpl_toolkits.mplot3d.art3d.Poly3DCollection object at 0x7fe9bc5d3430>, (7, 3, 4): <mpl_toolkits.mplot3d.art3d.Poly3DCollection object at 0x7fe9bc5d36a0>, (7, 3, 6): <mpl_toolkits.mplot3d.art3d.Poly3DCollection object at 0x7fe9bc5d3940>, (7, 4, 4): <mpl_toolkits.mplot3d.art3d.Poly3DCollection object at 0x7fe9bc5d3bb0>, (7, 4, 7): <mpl_toolkits.mplot3d.art3d.Poly3DCollection object at 0x7fe9bc5d3e20>, (7, 5, 5): <mpl_toolkits.mplot3d.art3d.Poly3DCollection object at 0x7fe9bc5d3fd0>, (7, 5, 8): <mpl_toolkits.mplot3d.art3d.Poly3DCollection object at 0x7fe9bc5e0340>, (7, 6, 5): <mpl_toolkits.mplot3d.art3d.Poly3DCollection object at 0x7fe9bc5e05b0>, (7, 6, 8): <mpl_toolkits.mplot3d.art3d.Poly3DCollection object at 0x7fe9bc5e0820>, (7, 7, 4): <mpl_toolkits.mplot3d.art3d.Poly3DCollection object at 0x7fe9bc5e0a90>, (7, 7, 7): <mpl_toolkits.mplot3d.art3d.Poly3DCollection object at 0x7fe9bc5e0d00>, (7, 8, 4): <mpl_toolkits.mplot3d.art3d.Poly3DCollection object at 0x7fe9bc5e0f70>, (7, 8, 6): <mpl_toolkits.mplot3d.art3d.Poly3DCollection object at 0x7fe9bc5f3220>, (7, 9, 3): <mpl_toolkits.mplot3d.art3d.Poly3DCollection object at 0x7fe9bc5f3490>, (7, 9, 5): <mpl_toolkits.mplot3d.art3d.Poly3DCollection object at 0x7fe9bc5f3700>, (7, 10, 2): <mpl_toolkits.mplot3d.art3d.Poly3DCollection object at 0x7fe9bc5f3970>, (7, 10, 5): <mpl_toolkits.mplot3d.art3d.Poly3DCollection object at 0x7fe9bc5f3be0>, (7, 11, 1): <mpl_toolkits.mplot3d.art3d.Poly3DCollection object at 0x7fe9bc5f3e50>, (7, 11, 4): <mpl_toolkits.mplot3d.art3d.Poly3DCollection object at 0x7fe9bc5f3fd0>, (7, 12, 1): <mpl_toolkits.mplot3d.art3d.Poly3DCollection object at 0x7fe9bc584370>, (7, 12, 4): <mpl_toolkits.mplot3d.art3d.Poly3DCollection object at 0x7fe9bc5845e0>, (7, 13, 2): <mpl_toolkits.mplot3d.art3d.Poly3DCollection object at 0x7fe9bc584850>, (7, 13, 5): <mpl_toolkits.mplot3d.art3d.Poly3DCollection object at 0x7fe9bc584ac0>, (7, 14, 3): <mpl_toolkits.mplot3d.art3d.Poly3DCollection object at 0x7fe9bc584d30>, (7, 14, 5): <mpl_toolkits.mplot3d.art3d.Poly3DCollection object at 0x7fe9bc584fa0>, (7, 15, 4): <mpl_toolkits.mplot3d.art3d.Poly3DCollection object at 0x7fe9bc592250>, (7, 15, 6): <mpl_toolkits.mplot3d.art3d.Poly3DCollection object at 0x7fe9bc5924c0>, (7, 16, 4): <mpl_toolkits.mplot3d.art3d.Poly3DCollection object at 0x7fe9bc592730>, (7, 16, 7): <mpl_toolkits.mplot3d.art3d.Poly3DCollection object at 0x7fe9bc5929a0>, (7, 17, 5): <mpl_toolkits.mplot3d.art3d.Poly3DCollection object at 0x7fe9bc592c10>, (7, 17, 8): <mpl_toolkits.mplot3d.art3d.Poly3DCollection object at 0x7fe9bc592e80>, (7, 18, 5): <mpl_toolkits.mplot3d.art3d.Poly3DCollection object at 0x7fe9bc5a4130>, (7, 18, 8): <mpl_toolkits.mplot3d.art3d.Poly3DCollection object at 0x7fe9bc5a43a0>, (7, 19, 4): <mpl_toolkits.mplot3d.art3d.Poly3DCollection object at 0x7fe9bc5a4610>, (7, 19, 7): <mpl_toolkits.mplot3d.art3d.Poly3DCollection object at 0x7fe9bc5a4880>, (7, 20, 4): <mpl_toolkits.mplot3d.art3d.Poly3DCollection object at 0x7fe9bc5a4af0>, (7, 20, 6): <mpl_toolkits.mplot3d.art3d.Poly3DCollection object at 0x7fe9bc5a4d60>, (7, 21, 3): <mpl_toolkits.mplot3d.art3d.Poly3DCollection object at 0x7fe9bc5a4fd0>, (7, 21, 5): <mpl_toolkits.mplot3d.art3d.Poly3DCollection object at 0x7fe9bc5b3280>, (7, 22, 2): <mpl_toolkits.mplot3d.art3d.Poly3DCollection object at 0x7fe9bc5b34f0>, (7, 22, 5): <mpl_toolkits.mplot3d.art3d.Poly3DCollection object at 0x7fe9bc5b3760>, (7, 23, 1): <mpl_toolkits.mplot3d.art3d.Poly3DCollection object at 0x7fe9bc5b39d0>, (7, 23, 4): <mpl_toolkits.mplot3d.art3d.Poly3DCollection object at 0x7fe9bc5b3c40>, (8, 0, 2): <mpl_toolkits.mplot3d.art3d.Poly3DCollection object at 0x7fe9bc5b3eb0>, (8, 0, 3): <mpl_toolkits.mplot3d.art3d.Poly3DCollection object at 0x7fe9bc543160>, (8, 1, 2): <mpl_toolkits.mplot3d.art3d.Poly3DCollection object at 0x7fe9bc5433d0>, (8, 1, 4): <mpl_toolkits.mplot3d.art3d.Poly3DCollection object at 0x7fe9bc543640>, (8, 2, 3): <mpl_toolkits.mplot3d.art3d.Poly3DCollection object at 0x7fe9bc5438b0>, (8, 2, 5): <mpl_toolkits.mplot3d.art3d.Poly3DCollection object at 0x7fe9bc543b20>, (8, 3, 4): <mpl_toolkits.mplot3d.art3d.Poly3DCollection object at 0x7fe9bc543d90>, (8, 3, 6): <mpl_toolkits.mplot3d.art3d.Poly3DCollection object at 0x7fe9bc543f40>, (8, 4, 5): <mpl_toolkits.mplot3d.art3d.Poly3DCollection object at 0x7fe9bc5542b0>, (8, 4, 7): <mpl_toolkits.mplot3d.art3d.Poly3DCollection object at 0x7fe9bc554520>, (8, 5, 6): <mpl_toolkits.mplot3d.art3d.Poly3DCollection object at 0x7fe9bc554790>, (8, 5, 7): <mpl_toolkits.mplot3d.art3d.Poly3DCollection object at 0x7fe9bc554a00>, (8, 6, 6): <mpl_toolkits.mplot3d.art3d.Poly3DCollection object at 0x7fe9bc554c70>, (8, 6, 7): <mpl_toolkits.mplot3d.art3d.Poly3DCollection object at 0x7fe9bc554ee0>, (8, 7, 5): <mpl_toolkits.mplot3d.art3d.Poly3DCollection object at 0x7fe9bc564190>, (8, 7, 7): <mpl_toolkits.mplot3d.art3d.Poly3DCollection object at 0x7fe9bc564400>, (8, 8, 4): <mpl_toolkits.mplot3d.art3d.Poly3DCollection object at 0x7fe9bc564670>, (8, 8, 6): <mpl_toolkits.mplot3d.art3d.Poly3DCollection object at 0x7fe9bc5648e0>, (8, 9, 3): <mpl_toolkits.mplot3d.art3d.Poly3DCollection object at 0x7fe9bc564b50>, (8, 9, 5): <mpl_toolkits.mplot3d.art3d.Poly3DCollection object at 0x7fe9bc564dc0>, (8, 10, 2): <mpl_toolkits.mplot3d.art3d.Poly3DCollection object at 0x7fe9bc564f70>, (8, 10, 4): <mpl_toolkits.mplot3d.art3d.Poly3DCollection object at 0x7fe9bc5742e0>, (8, 11, 2): <mpl_toolkits.mplot3d.art3d.Poly3DCollection object at 0x7fe9bc574550>, (8, 11, 3): <mpl_toolkits.mplot3d.art3d.Poly3DCollection object at 0x7fe9bc5747c0>, (8, 12, 2): <mpl_toolkits.mplot3d.art3d.Poly3DCollection object at 0x7fe9bc574a30>, (8, 12, 3): <mpl_toolkits.mplot3d.art3d.Poly3DCollection object at 0x7fe9bc574ca0>, (8, 13, 2): <mpl_toolkits.mplot3d.art3d.Poly3DCollection object at 0x7fe9bc574f10>, (8, 13, 4): <mpl_toolkits.mplot3d.art3d.Poly3DCollection object at 0x7fe9bc5021c0>, (8, 14, 3): <mpl_toolkits.mplot3d.art3d.Poly3DCollection object at 0x7fe9bc502430>, (8, 14, 5): <mpl_toolkits.mplot3d.art3d.Poly3DCollection object at 0x7fe9bc5026a0>, (8, 15, 4): <mpl_toolkits.mplot3d.art3d.Poly3DCollection object at 0x7fe9bc502910>, (8, 15, 6): <mpl_toolkits.mplot3d.art3d.Poly3DCollection object at 0x7fe9bc502b80>, (8, 16, 5): <mpl_toolkits.mplot3d.art3d.Poly3DCollection object at 0x7fe9bc502df0>, (8, 16, 7): <mpl_toolkits.mplot3d.art3d.Poly3DCollection object at 0x7fe9bc502fa0>, (8, 17, 6): <mpl_toolkits.mplot3d.art3d.Poly3DCollection object at 0x7fe9bc515310>, (8, 17, 7): <mpl_toolkits.mplot3d.art3d.Poly3DCollection object at 0x7fe9bc515580>, (8, 18, 6): <mpl_toolkits.mplot3d.art3d.Poly3DCollection object at 0x7fe9bc5157f0>, (8, 18, 7): <mpl_toolkits.mplot3d.art3d.Poly3DCollection object at 0x7fe9bc515a60>, (8, 19, 5): <mpl_toolkits.mplot3d.art3d.Poly3DCollection object at 0x7fe9bc515cd0>, (8, 19, 7): <mpl_toolkits.mplot3d.art3d.Poly3DCollection object at 0x7fe9bc515f40>, (8, 20, 4): <mpl_toolkits.mplot3d.art3d.Poly3DCollection object at 0x7fe9bc5261f0>, (8, 20, 6): <mpl_toolkits.mplot3d.art3d.Poly3DCollection object at 0x7fe9bc526460>, (8, 21, 3): <mpl_toolkits.mplot3d.art3d.Poly3DCollection object at 0x7fe9bc5266d0>, (8, 21, 5): <mpl_toolkits.mplot3d.art3d.Poly3DCollection object at 0x7fe9bc526940>, (8, 22, 2): <mpl_toolkits.mplot3d.art3d.Poly3DCollection object at 0x7fe9bc526bb0>, (8, 22, 4): <mpl_toolkits.mplot3d.art3d.Poly3DCollection object at 0x7fe9bc526e20>, (8, 23, 2): <mpl_toolkits.mplot3d.art3d.Poly3DCollection object at 0x7fe9bc526fd0>, (8, 23, 3): <mpl_toolkits.mplot3d.art3d.Poly3DCollection object at 0x7fe9bc535340>, (5, 1, 3): <mpl_toolkits.mplot3d.art3d.Poly3DCollection object at 0x7fe9bc5355b0>, (8, 1, 3): <mpl_toolkits.mplot3d.art3d.Poly3DCollection object at 0x7fe9bc535820>, (5, 2, 4): <mpl_toolkits.mplot3d.art3d.Poly3DCollection object at 0x7fe9bc535a90>, (8, 2, 4): <mpl_toolkits.mplot3d.art3d.Poly3DCollection object at 0x7fe9bc535d00>, (5, 3, 5): <mpl_toolkits.mplot3d.art3d.Poly3DCollection object at 0x7fe9bc535f70>, (8, 3, 5): <mpl_toolkits.mplot3d.art3d.Poly3DCollection object at 0x7fe9bc4c5220>, (5, 4, 6): <mpl_toolkits.mplot3d.art3d.Poly3DCollection object at 0x7fe9bc4c54c0>, (8, 4, 6): <mpl_toolkits.mplot3d.art3d.Poly3DCollection object at 0x7fe9bc4c5730>, (5, 7, 6): <mpl_toolkits.mplot3d.art3d.Poly3DCollection object at 0x7fe9bc4c59a0>, (8, 7, 6): <mpl_toolkits.mplot3d.art3d.Poly3DCollection object at 0x7fe9bc4c5c10>, (5, 8, 5): <mpl_toolkits.mplot3d.art3d.Poly3DCollection object at 0x7fe9bc4c5e80>, (8, 8, 5): <mpl_toolkits.mplot3d.art3d.Poly3DCollection object at 0x7fe9bc4d6130>, (5, 9, 4): <mpl_toolkits.mplot3d.art3d.Poly3DCollection object at 0x7fe9bc4d63a0>, (8, 9, 4): <mpl_toolkits.mplot3d.art3d.Poly3DCollection object at 0x7fe9bc4d6610>, (5, 10, 3): <mpl_toolkits.mplot3d.art3d.Poly3DCollection object at 0x7fe9bc4d6880>, (8, 10, 3): <mpl_toolkits.mplot3d.art3d.Poly3DCollection object at 0x7fe9bc4d6af0>, (5, 13, 3): <mpl_toolkits.mplot3d.art3d.Poly3DCollection object at 0x7fe9bc4d6d60>, (8, 13, 3): <mpl_toolkits.mplot3d.art3d.Poly3DCollection object at 0x7fe9bc4d6fd0>, (5, 14, 4): <mpl_toolkits.mplot3d.art3d.Poly3DCollection object at 0x7fe9bc4e5280>, (8, 14, 4): <mpl_toolkits.mplot3d.art3d.Poly3DCollection object at 0x7fe9bc4e54f0>, (5, 15, 5): <mpl_toolkits.mplot3d.art3d.Poly3DCollection object at 0x7fe9bc4e5760>, (8, 15, 5): <mpl_toolkits.mplot3d.art3d.Poly3DCollection object at 0x7fe9bc4e59d0>, (5, 16, 6): <mpl_toolkits.mplot3d.art3d.Poly3DCollection object at 0x7fe9bc4e5c40>, (8, 16, 6): <mpl_toolkits.mplot3d.art3d.Poly3DCollection object at 0x7fe9bc4e5eb0>, (5, 19, 6): <mpl_toolkits.mplot3d.art3d.Poly3DCollection object at 0x7fe9bc4f6160>, (8, 19, 6): <mpl_toolkits.mplot3d.art3d.Poly3DCollection object at 0x7fe9bc4f63d0>, (5, 20, 5): <mpl_toolkits.mplot3d.art3d.Poly3DCollection object at 0x7fe9bc4f6640>, (8, 20, 5): <mpl_toolkits.mplot3d.art3d.Poly3DCollection object at 0x7fe9bc4f68b0>, (5, 21, 4): <mpl_toolkits.mplot3d.art3d.Poly3DCollection object at 0x7fe9bc4f6b20>, (8, 21, 4): <mpl_toolkits.mplot3d.art3d.Poly3DCollection object at 0x7fe9bc4f6d90>, (5, 22, 3): <mpl_toolkits.mplot3d.art3d.Poly3DCollection object at 0x7fe9bc4f6f70>, (8, 22, 3): <mpl_toolkits.mplot3d.art3d.Poly3DCollection object at 0x7fe9bc4852e0>, (6, 0, 2): <mpl_toolkits.mplot3d.art3d.Poly3DCollection object at 0x7fe9bc485550>, (6, 23, 2): <mpl_toolkits.mplot3d.art3d.Poly3DCollection object at 0x7fe9bc4857c0>, (7, 0, 2): <mpl_toolkits.mplot3d.art3d.Poly3DCollection object at 0x7fe9bc485a30>, (7, 23, 2): <mpl_toolkits.mplot3d.art3d.Poly3DCollection object at 0x7fe9bc485ca0>, (6, 0, 3): <mpl_toolkits.mplot3d.art3d.Poly3DCollection object at 0x7fe9bc485f10>, (6, 23, 3): <mpl_toolkits.mplot3d.art3d.Poly3DCollection object at 0x7fe9bc4971c0>, (7, 0, 3): <mpl_toolkits.mplot3d.art3d.Poly3DCollection object at 0x7fe9bc497430>, (7, 23, 3): <mpl_toolkits.mplot3d.art3d.Poly3DCollection object at 0x7fe9bc4976a0>}
plt.show()
Those blocks are called voxels. If you have seen reservoir simulation you know what they represent. We could also have plot this in cartesian coordinates instead of cylindrical.
Example 3
This is a simpler example of the blocks in cylindrical coordinates above.
Why is this important?
If we will be applying machine learning algorithms we need to have some tools outside the conventional reservoir simulators that do not have machine learning or artificial intelligence capabilities. This means, if you have the reservoir grid plus the saturations, permeability and wells location, it very likely they will be in text readable format. At least, in Eclipse they are. Then, you will able to transform the grid and reservoir properties using your production history and achieve more accurate and faster matching. There is abundant literature on the subject in the SPE papers.
# https://matplotlib.org/gallery/mplot3d/voxels_numpy_logo.html
import matplotlib.pyplot as plt
import numpy as np
# This import registers the 3D projection, but is otherwise unused.
from mpl_toolkits.mplot3d import Axes3D # noqa: F401 unused import
def explode(data):
size = np.array(data.shape)*2
data_e = np.zeros(size - 1, dtype=data.dtype)
data_e[::2, ::2, ::2] = data
return data_e
# build up the numpy logo
n_voxels = np.zeros((4, 3, 4), dtype=bool)
n_voxels[0, 0, :] = True
n_voxels[-1, 0, :] = True
n_voxels[1, 0, 2] = True
n_voxels[2, 0, 1] = True
facecolors = np.where(n_voxels, '#FFD65DC0', '#7A88CCC0')
edgecolors = np.where(n_voxels, '#BFAB6E', '#7D84A6')
filled = np.ones(n_voxels.shape)
# upscale the above voxel image, leaving gaps
filled_2 = explode(filled)
fcolors_2 = explode(facecolors)
ecolors_2 = explode(edgecolors)
# Shrink the gaps
x, y, z = np.indices(np.array(filled_2.shape) + 1).astype(float) // 2
x[0::2, :, :] += 0.05
y[:, 0::2, :] += 0.05
z[:, :, 0::2] += 0.05
x[1::2, :, :] += 0.95
y[:, 1::2, :] += 0.95
z[:, :, 1::2] += 0.95
fig = plt.figure()
ax = fig.gca(projection='3d')
ax.voxels(x, y, z, filled_2, facecolors=fcolors_2, edgecolors=ecolors_2)
#> {(0, 0, 0): <mpl_toolkits.mplot3d.art3d.Poly3DCollection object at 0x7fe9bc400370>, (0, 0, 2): <mpl_toolkits.mplot3d.art3d.Poly3DCollection object at 0x7fe9bc400580>, (0, 0, 4): <mpl_toolkits.mplot3d.art3d.Poly3DCollection object at 0x7fe9bc4007f0>, (0, 0, 6): <mpl_toolkits.mplot3d.art3d.Poly3DCollection object at 0x7fe9bc400a30>, (0, 2, 0): <mpl_toolkits.mplot3d.art3d.Poly3DCollection object at 0x7fe9bc400ca0>, (0, 2, 2): <mpl_toolkits.mplot3d.art3d.Poly3DCollection object at 0x7fe9bc400f10>, (0, 2, 4): <mpl_toolkits.mplot3d.art3d.Poly3DCollection object at 0x7fe9bc3981c0>, (0, 2, 6): <mpl_toolkits.mplot3d.art3d.Poly3DCollection object at 0x7fe9bc398430>, (0, 4, 0): <mpl_toolkits.mplot3d.art3d.Poly3DCollection object at 0x7fe9bc3986d0>, (0, 4, 2): <mpl_toolkits.mplot3d.art3d.Poly3DCollection object at 0x7fe9bc398940>, (0, 4, 4): <mpl_toolkits.mplot3d.art3d.Poly3DCollection object at 0x7fe9bc398bb0>, (0, 4, 6): <mpl_toolkits.mplot3d.art3d.Poly3DCollection object at 0x7fe9bc398e20>, (2, 0, 0): <mpl_toolkits.mplot3d.art3d.Poly3DCollection object at 0x7fe9bc398fd0>, (2, 0, 2): <mpl_toolkits.mplot3d.art3d.Poly3DCollection object at 0x7fe9bc3a8370>, (2, 0, 4): <mpl_toolkits.mplot3d.art3d.Poly3DCollection object at 0x7fe9bc3a8610>, (2, 0, 6): <mpl_toolkits.mplot3d.art3d.Poly3DCollection object at 0x7fe9bc3a8880>, (2, 2, 0): <mpl_toolkits.mplot3d.art3d.Poly3DCollection object at 0x7fe9bc3a8af0>, (2, 2, 2): <mpl_toolkits.mplot3d.art3d.Poly3DCollection object at 0x7fe9bc3a8d60>, (2, 2, 4): <mpl_toolkits.mplot3d.art3d.Poly3DCollection object at 0x7fe9bc3a8fd0>, (2, 2, 6): <mpl_toolkits.mplot3d.art3d.Poly3DCollection object at 0x7fe9bc3ba280>, (2, 4, 0): <mpl_toolkits.mplot3d.art3d.Poly3DCollection object at 0x7fe9bc3ba4f0>, (2, 4, 2): <mpl_toolkits.mplot3d.art3d.Poly3DCollection object at 0x7fe9bc3ba760>, (2, 4, 4): <mpl_toolkits.mplot3d.art3d.Poly3DCollection object at 0x7fe9bc3ba9d0>, (2, 4, 6): <mpl_toolkits.mplot3d.art3d.Poly3DCollection object at 0x7fe9bc3bac40>, (4, 0, 0): <mpl_toolkits.mplot3d.art3d.Poly3DCollection object at 0x7fe9bc3baee0>, (4, 0, 2): <mpl_toolkits.mplot3d.art3d.Poly3DCollection object at 0x7fe9bc34b190>, (4, 0, 4): <mpl_toolkits.mplot3d.art3d.Poly3DCollection object at 0x7fe9bc34b400>, (4, 0, 6): <mpl_toolkits.mplot3d.art3d.Poly3DCollection object at 0x7fe9bc34b670>, (4, 2, 0): <mpl_toolkits.mplot3d.art3d.Poly3DCollection object at 0x7fe9bc34b8e0>, (4, 2, 2): <mpl_toolkits.mplot3d.art3d.Poly3DCollection object at 0x7fe9bc34bb50>, (4, 2, 4): <mpl_toolkits.mplot3d.art3d.Poly3DCollection object at 0x7fe9bc34bdc0>, (4, 2, 6): <mpl_toolkits.mplot3d.art3d.Poly3DCollection object at 0x7fe9bc34bf70>, (4, 4, 0): <mpl_toolkits.mplot3d.art3d.Poly3DCollection object at 0x7fe9bc3582e0>, (4, 4, 2): <mpl_toolkits.mplot3d.art3d.Poly3DCollection object at 0x7fe9bc358550>, (4, 4, 4): <mpl_toolkits.mplot3d.art3d.Poly3DCollection object at 0x7fe9bc3587c0>, (4, 4, 6): <mpl_toolkits.mplot3d.art3d.Poly3DCollection object at 0x7fe9bc358a30>, (6, 0, 0): <mpl_toolkits.mplot3d.art3d.Poly3DCollection object at 0x7fe9bc358ca0>, (6, 0, 2): <mpl_toolkits.mplot3d.art3d.Poly3DCollection object at 0x7fe9bc358f10>, (6, 0, 4): <mpl_toolkits.mplot3d.art3d.Poly3DCollection object at 0x7fe9bc36b1c0>, (6, 0, 6): <mpl_toolkits.mplot3d.art3d.Poly3DCollection object at 0x7fe9bc36b430>, (6, 2, 0): <mpl_toolkits.mplot3d.art3d.Poly3DCollection object at 0x7fe9bc36b6a0>, (6, 2, 2): <mpl_toolkits.mplot3d.art3d.Poly3DCollection object at 0x7fe9bc36b910>, (6, 2, 4): <mpl_toolkits.mplot3d.art3d.Poly3DCollection object at 0x7fe9bc36bb80>, (6, 2, 6): <mpl_toolkits.mplot3d.art3d.Poly3DCollection object at 0x7fe9bc36bdf0>, (6, 4, 0): <mpl_toolkits.mplot3d.art3d.Poly3DCollection object at 0x7fe9bc36bfa0>, (6, 4, 2): <mpl_toolkits.mplot3d.art3d.Poly3DCollection object at 0x7fe9bc37a310>, (6, 4, 4): <mpl_toolkits.mplot3d.art3d.Poly3DCollection object at 0x7fe9bc37a580>, (6, 4, 6): <mpl_toolkits.mplot3d.art3d.Poly3DCollection object at 0x7fe9bc37a7f0>}
plt.show()
Why Python and R?
I know what you are thinking. If Python has all these plotting capabilities, why not doing all the coding just with Python?
Because:
- R is much better and faster at data manipulation
- In R is easier to use C, C++ and Fortran libraries for matrix and array operations
- Report generation in R is publishing quality.
- You can use embedded Latex, Beamer, tikz, and innumerable packages for equations, presentations, diagrams that belong to the universe of reproducibility in data science.
And the best reason of all: We will be ahead of the pack by using both powerful scripting languages: Python and R.
And with the Volve datasets now available the task will be more productive.
Warning
This page is dynamic, it will be changing in the next hours until the edition is complete. In the meantime, enjoy.
Code
All the code will be available in GitHub at https://github.com/f0nzie/
References
Integrated Development Environment↩︎